
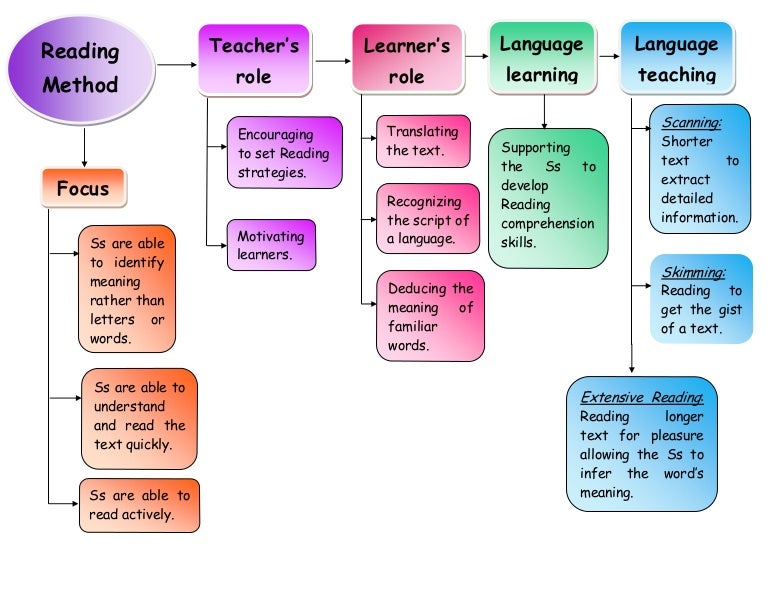
Now, if we look at the 1-D mapping based on the Euclidean metric, we see that for points which are far apart(a & b) have been mapped poorly. We will reduce the data to 1-D using the Euclidean distances and approximate geodesic distances. Why geodesic distances are better than the Euclidean distances in nonlinear manifolds? Suppose our data lies on a circular manifold in a 2-D structure like in the image below. Let's understand this with an extremely simple 2-D example. In contrast to this, if we measure the distance between two points by following the manifold, we will have a better approximation of how far or near two points are. If neighborhood contains holes, then Euclidean distances can be highly misleading. In non-linear manifolds, the Euclidean metric for distance holds good if and only if neighborhood structure can be approximated as linear. And then, through eigenvalue decomposition of the geodesic distance matrix, it finds the low dimensional embedding of the dataset. After that, it uses graph distance to the approximate geodesic distance between all pairs of points. Isomap starts by creating a neighborhood network. Isomap is a non-linear dimensionality reduction method based on the spectral theory which tries to preserve the geodesic distances in the lower dimension. (A jupyter notebook with math and code(spark) is available on github repo ) Diving Deeper into Dimension Reduction with Independent Components Analysis (ICA).Understanding Dimension Reduction with Principal Component Analysis (PCA).
#Iso method map series
This tutorial is from a 7 part series on Dimension Reduction:
